Transform images with Flux Kontext
In this example, we run the Flux Kontext model in image-to-image mode: the model takes in a prompt and an image and transforms the image to better match the prompt.
For example, the model transformed the first image into the second based on the prompt βA cute dog wizard inspired by Gandalf from Lord of the Rings, featuring detailed fantasy elements in Studio Ghibli styleβ.
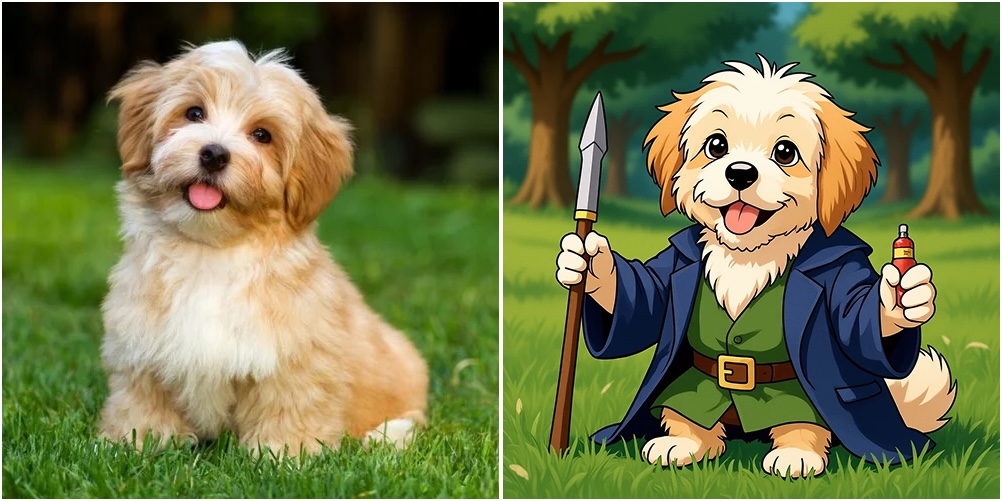
The model is Black Forest Labsβ FLUX.1-Kontext-dev. Learn more about the model here.
Define a container image
First, we define the environment the model inference will run in, the container image.
from io import BytesIO
from pathlib import Path
import modal
diffusers_commit_sha = "00f95b9755718aabb65456e791b8408526ae6e76"
image = (
modal.Image.debian_slim(python_version="3.12")
.apt_install("git")
.pip_install(
"accelerate~=1.8.1", # Allows `device_map="balanced"``, for computation of optimized device_map
f"git+https://github.com/huggingface/diffusers.git@{diffusers_commit_sha}", # Provides model libraries
"huggingface-hub[hf-transfer]~=0.33.1", # Lets us download models from Hugging Face's Hub
"Pillow~=11.2.1", # Image manipulation in Python
"safetensors~=0.5.3", # Enables safetensor format as opposed to using unsafe pickle format
"transformers~=4.53.0",
"sentencepiece~=0.2.0",
"torch==2.7.1",
extra_index_url="https://download.pytorch.org/whl/cu128",
)
)
MODEL_NAME = "black-forest-labs/FLUX.1-Kontext-dev"
CACHE_DIR = Path("/cache")
cache_volume = modal.Volume.from_name("hf-hub-cache", create_if_missing=True)
volumes = {CACHE_DIR: cache_volume}
secrets = [modal.Secret.from_name("huggingface-secret")]
image = image.env(
{
"HF_HUB_ENABLE_HF_TRANSFER": "1", # Allows faster model downloads
"HF_HOME": str(CACHE_DIR), # Points the Hugging Face cache to a Volume
}
)
app = modal.App("image-to-image")
with image.imports():
import torch
from diffusers import FluxKontextPipeline
from diffusers.utils import load_image
from PIL import Image
Setting up and running Flux Kontext
The Modal Cls
defined below contains all the logic to set up and run Flux Kontext.
The container lifecycle decorator
(@modal.enter()
) ensures that the model is loaded into memory when a container starts, before it picks up any inputs.
The inference
method runs the actual model inference. It takes in an image as a collection of bytes
and a string prompt
and returns
a new image (also as a collection of bytes
).
To avoid excessive cold-starts, we set the scaledown_window
to 240 seconds, meaning once a GPU has loaded the model it will stay
online for 4 minutes before spinning down.
@app.cls(
image=image, gpu="B200", volumes=volumes, secrets=secrets, scaledown_window=240
)
class Model:
@modal.enter()
def enter(self):
print(f"Downloading {MODEL_NAME} if necessary...")
self.pipe = FluxKontextPipeline.from_pretrained(
MODEL_NAME,
revision="f9fdd1a95e0dfd7653cb0966cda2486745122695",
torch_dtype=torch.bfloat16,
device_map="balanced",
cache_dir=CACHE_DIR,
)
@modal.method()
def inference(
self, image_bytes: bytes, prompt: str, guidance_scale: float = 2.5
) -> bytes:
init_image = load_image(Image.open(BytesIO(image_bytes))).resize((512, 512))
image = self.pipe(
image=init_image,
prompt=prompt,
guidance_scale=guidance_scale,
generator=torch.Generator().manual_seed(42),
).images[0]
byte_stream = BytesIO()
image.save(byte_stream, format="PNG")
image_bytes = byte_stream.getvalue()
return image_bytes
Running the model from the command line
You can run the model from the command line with
modal run image_to_image.py
Use --help
for additional details.
@app.local_entrypoint()
def main(
image_path=Path(__file__).parent / "demo_images/dog.png",
prompt="A cute dog wizard inspired by Gandalf from Lord of the Rings, featuring detailed fantasy elements in Studio Ghibli style",
strength=0.9, # increase to favor the prompt over the baseline image
):
print(f"π¨ reading input image from {image_path}")
input_image_bytes = Path(image_path).read_bytes()
print(f"π¨ editing image with prompt {prompt}")
output_image_bytes = Model().inference.remote(input_image_bytes, prompt)
dir = Path("/tmp/stable-diffusion")
dir.mkdir(exist_ok=True, parents=True)
output_path = dir / "output.png"
print(f"π¨ saving output image to {output_path}")
output_path.write_bytes(output_image_bytes)