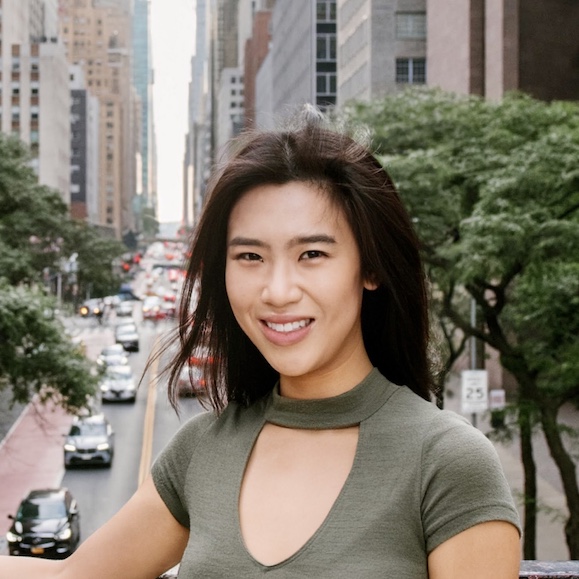
Introduction to Stable Diffusion XL
Stable Diffusion XL (SDXL) generates images of high quality in virtually any art style and is the best open model for photorealism. It was trained on 1024x1024 images, and it is suitable for generating images with those resolutions.
Why should you run Stable Diffusion XL on Modal?
Modal is the best and easiest way to access a GPU for running models like Stable Diffusion XL. With Modal, you just write a Python function, apply a decorator, and deploy.
This flexibility means that you can also easily fine-tune Stable Diffusion XL, store the LoRA weights, and serve them as a web service, all on Modal infrastructure.
Example code for running the Stable Diffusion XL image generation model on Modal
To run the following code, you will need to:
- Create an account at modal.com
- Run
pip install modal
to install the modal Python package - Run
modal setup
to authenticate (if this doesn’t work, trypython -m modal setup
) - Copy the code below into a file called
app.py
- Run
modal run app.py
import io
import random
from pathlib import Path
import modal
app = modal.App("stable-diffusion-xl-model-library")
image = (
modal.Image.debian_slim(python_version="3.12")
.pip_install(
"accelerate==0.33.0",
"diffusers==0.31.0",
"fastapi[standard]==0.115.4",
"huggingface-hub[hf_transfer]==0.25.2",
"sentencepiece==0.2.0",
"torch==2.5.1",
"torchvision==0.20.1",
"transformers~=4.44.0",
)
.env({"HF_HUB_ENABLE_HF_TRANSFER": "1"})
)
with image.imports():
import torch
import diffusers
CACHE_DIR = "/cache"
cache_vol = modal.Volume.from_name("hf-hub-cache", create_if_missing=True)
@app.cls(
image=image,
gpu="H100",
volumes={CACHE_DIR: cache_vol},
timeout=600,
)
class Inference:
@modal.enter()
def initialize(self):
self.pipe = diffusers.DiffusionPipeline.from_pretrained(
"stabilityai/stable-diffusion-xl-base-1.0",
cache_dir=CACHE_DIR,
torch_dtype=torch.float16,
use_safetensors=True,
variant="fp16",
)
@modal.enter()
def move_to_gpu(self):
self.pipe.to("cuda")
@modal.method()
def run(self, prompt: str, batch_size: int = 4, seed: int = None) -> list[bytes]:
seed = seed if seed is not None else random.randint(0, 2**32 - 1)
print("seeding RNG with", seed)
torch.manual_seed(seed)
images = self.pipe(
prompt,
).images
image_output = []
for image in images:
with io.BytesIO() as buf:
image.save(buf, format="PNG")
image_output.append(buf.getvalue())
torch.cuda.empty_cache()
return image_output
@app.local_entrypoint()
def main(prompt: str = "A princess riding on a pony"):
output_dir = Path("/tmp/stable-diffusion")
output_dir.mkdir(exist_ok=True)
images = Inference().run.remote(prompt, batch_size=1)
for i, image_bytes in enumerate(images):
output_path = output_dir / f"output_{i:02d}.png"
output_path.write_bytes(image_bytes)
print(f"Saved {output_path}")